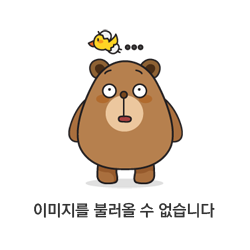
오랜만에 논문 리뷰! 대학원 생활을 하면서 너무 정신이 없어서 글을 쓸 생각을 못하면서 한동안 지냈다. 연휴 기간 동안 어느정도 여유가 생겨서 오랜만에 해보는 논문 리뷰!
요즘은 prompting 관련한 연구를 연구실에서 진행 중이다. 간단하게 정리해보면, 엄청 많은 텍스트 데이터를 기반으로 학습한 Pre-trained Language Model 들을 (예를들어, BERT, GPT2, RoBERTa 등등) 이용해서 어떠한 downstream task를 풀 때 기본적으로 모델의 모든 파라미터를 학습시키는 fine-tuning 방식을 가장 많이 사용했었다. 이후에 GPT-3 가 공개되면서, in-context learning 이 실제로 큰 효과가 있다는 것을 보였고, 이를 기반으로 "prompting"이라는 연구가 발전되었다. 정말 정말 간단하게 설명하면, 기존의 fine-tuning처럼 모델의 전체 파라미터를 학습하는게 아니라, 모델의 파라미터는 그대로 유지하면서 모델에게 우리가 풀고자하는 downstream task에 대한 설명을 주는 것이다. 그러면 모델을 그 설명을 보고 지금 어떠한 문제를 풀려고하는지 이해하고, 입력으로 주어진 문장까지 이해해서 적절한 답변을 제공하는 방식이다. 이 모든 과정을 PLM들이 이미 충분히 많은 데이터로 학습이 되어있고, 자연어를 어느정도 잘 이해하고 있다는 가정을 기반으로 하고 있다. 이러한 과정에서 우리가 모델에게 제공하는 문제의 설명(?)을 prompt라고 이해하면 될 것 같다.
사실 이 이후에는 Prompt를 기반으로 fine-tuning을 하면 더 효과적이라는 prompt-based fine-tuning이라던지, 자연어로 제공되는 prompt가 모델이 이해하기에는 optimal 하지 않을 수 있기 때문에 학습가능한 trainable continuous prompt를 학습하는 연구(이게 사실 요즘 대부분 prompting 연구의 주 방향이긴 함) 등으로 나아가고 있다.
이 이야기가 왜 이번 논문하고 무슨 관계가 있느냐! 하면, 학생 입장에서 이러한 대현 PLM들을 새로 만들거나 하는건 사실상 불가능하다. 큰 회사나 기업들이 만들어낸 PLM들을 최대한 잘 활용하는게 중요한데 (prompting도 이러한 방향 중 하나), prompting을 연구하면서 가장 많이 든 생각이 이 모델이 이 문제를 해결할 능력이 없다면 우리가 아무리 좋은 prompt를 만들어서 줘도 쓸모 없는게 아닌가? 였다. 이런 고민을 계속 하면서 점점 PLM안에 내제되어 있는 능력이 뭔지, 지식은 어느정도 가지고 있는지, 어떠한 예측을 하는데 어떤 요소들을 기반으로 이러한 예측을 했는지 등 PLM을 이해하는 것도 매우 중요할 것 같다는 생각이 들었다. 그래서~ 이번에는 뭔가 NLP쪽 분야에서 Explainability를 다룬 서베이 논문을 가져와봤다. 나도 그냥 어떤 연구들이 진행되고 있는지 쭉 둘러보기 위해서 읽어봤고, 논문 자체에는 큰 내용이 없다. 그냥 참고할만한 논문들, 연구들의 큰 방향들에 대한 감을 잡아볼 수 있지 않을까 해서 가져와봤다.
- Local Interpretations for Explainable Natural Language Processing: A Survey
- Siwen Luo, Hamish Ivison, Caren Han, Josiah Poon
- arXiv 2021
Introduction
Deep Learining 모델들은 대부분 black-box 모델들이다. 입력을 주면 결과를 뱉어내지만, 이 결과를 도출하기까지 어떠한 연산이 이루어지는지, 어떠한 근거를 기반으로 이러한 결과를 제공하는지에 대한 정보를 알 수 없다. 아직은 인공지능이 많은 분야에 사용되고 있지는 않지만 (점점 늘어나고 있긴 함), 인공지능이 중요한 의사결정을 하는데 사용이 된다면 인공지능이 왜 이러한 결정을 내렸는지에 대한 이해는 필수적일 것이다. 항상 100% 정확한 판단을 하지 못하고, 정확한 판단을 했다고 하더라도 이러한 결정에 대한 근거와 설명이 필수적이 상황들이 많다. 시간이 지남에 따라 인공지능에 대한 explanation 이 점점 더 중요한 요소로 자리잡게 될 것이다.
- Peeking inside the black-box: A survey on Explainable Artificial Intelligence (XAI)
- Considerations for Evaluation and Generalization in Interpretable Machine Learning.
- A survey of methods for explaining black box models.
- Towards Faithfully Interpretable NLP Systems: How Should We Define and Evaluate Faithfulness?
Aspects of Interpretability
Interpretability 의 정의
아직까지 intrepretability에 대한 명확한 정의는 없다. 이 섹션에서는 interpretability 와 관련된 용어들을 간단하게 정리한다.
1. Explainability VS Interpreatbility : 두 용어 모두 동일한 의미로 사용된다 : 인공지능이 제공한 결정에 대한 설명이나 reasoning을 제공하는 능력. 차이점이라 하면, Interpreatbility 는 ML 커뮤니티에서 더 많이 사용되고, explainability는 HCI 커뮤니티에서 더 많이 사용된다는 정도?
The ability [of a model] to explain or to present [its predictions] in understandable terms to a human.
2. Local VS Global interpretability : Local interpretability의 경우 특정한 입력이 주어졌을 때, 해당 입력에 대한 예측에 대한 interpretability를 제공하는 것이고, gloabl interpretability의 경우는 어떠한 결정을 내리는데 모델 내부에서 이루어지는 전체 로직에 대한 설명이 가능한 경우를 의미한다. (대표적으로 decision tree)
3. Post-hoc VS In-built Interpretation : Post-hoc의 경우는 특정한 입력을 모델이 받아서 결과를 제공하고, 결과를 제공한 이후에 적용되는 방식이다. 이 방식은 model-agnostic하고, 모델이 예측하는 과정에 개입하지 않기 때문에 perfomance 에 영향을 주지 않는다. 대표적으로는 LIME이 있다. In-built 방식을 이름 그대로 예측하는 과정에 intrepretation 이 개입하는 방식이다.
- " Why should I trust you?" Explaining the predictions of any classifier.
- How much should you ask? On the question structure in QA systems.
Interpretability Requirements
Interpretability를 제공하는 다양한 방법들이 있는데, 이런 방법들은 각자 적합한 세팅이나 환경 등이 있다. 그러므로 각자의 상황에 알맞는 interpretability를 가져다가 사용해야 하는데, 이 때 고려해야 하는 사항들 몇 가지만 정리해보면 :
1. 특정 입력에 대한 설명이 필요한지(local interpretation), 아니면 모델의 전체 동작 방식에 대한 이해가 필요한지(global interpretation) ?
2. 설명을 받아보기까지 어느정도의 시간이 가용한지? 결과에 대한 설명이 빠르게 제공되어야 하는 경우도 있고 아닌 경우도 있으니..
3. 설명을 받아보는 사람의 전문성(?). ML background가 있는 사람과 아예 없는 사람에게는 다른 설명을 제공해야 하기 때문이다.
Dimensions of Interpretability
Interpretability는 다양한 측면에서 측정될 수 있는데, 이 논문에서는 4가지 요소로 구분에서 설명한다.
1. Faithfulness : interpretation 방식이 실제 reasoning 과정과 관련성이 높은지(?) Heatmap이나 attention map등
- A unified approach to interpreting model predictions
2. Stability : 비슷한 입력에 대해서 비슷한 결과를 제공하면 stable 한 interpretation method 라고 할 수 있다.
3. Comprehensibility : 제공된 설명을 사용자가 이해할 수 있는지?
4. Trustworthiness : 사용자가 이 방법론은 믿을 수 있는지? 예측하는 과정에 실수가 있어도, 실수에 대한 reasoning이 타당하다면 trustworthy하다고 할 수 있다. Acccuracy와 Faithfulness와는 다름.
Interpretability Methods
이 섹션에서는 실제로 다양한 interpretation method들에 대해서 다룬다. 크게 4가지 그룹으로 나눠서 설명한다 : rationale extraction, input perturbation, attribution method, attention weight extraction
1. Rationale Extraction
- Rationalizing Neural Predictions.
- Rethinking Cooperative Rationalization: Introspective Extraction and Complement Control.
2. Input Perturbation : 입력의 일부를 바꿔서 모델의 입력으로 줬을 때, 성능이 급격히 떨어지는 경우 바뀐 입력이 결정을 내리는데 매우 중요한 요소라는 것.
- HotFlip: White-Box Adversarial Examples for Text Classification.
- Anchors: High-precision model-agnostic explanations.
3. Attribution method : 결과에 대한 interpretation 을 gradient 를 보고 해석하는 방법. 한 개의 다른 feature만 있는 두 개의 입력을 사용할 때 큰 gradient 차이가 생긴다면 해당 feature는 결과 예측에 중요한 요소라고 생각한다.
- On pixel-wise explanations for non-linear classifier decisions by layer-wise relevance propagation
- On attribution of recurrent neural network predictions via additive decomposition.
- Learning important features through propagating activation differences.
4. Attention Weight Extraction : Attention mechanism을 사용하는 모델들에 적용될 수 있는 방법. 입력으로 주어진 token 중 높은 attention weight을 보이는 token이 중요한 token으로 이해한다. Attention 이 과연 interpretation을 하는데 있어서 중요한 역할을 하는지에 대해서는 아직 이야기가 많은 듯 하다.
- Towards Understanding Neural Machine Translation with Word Importance.
- Attention is not Explanation.
- AspectBased Sentiment Classification with Attentive Neural Turing Machines.
- Axiomatic attribution for deep networks.
- Attention is not not Explanation
- Deep modular co-attention networks for visual question answering.
Natural Language Explanation (NLE)
모델이 예측한 결과에 대한 설명을 사람이 이해할 수 있는 natural language 로 생성해서 제공하는 방법이다. VQA(Visual Question-Answering)에서 많이 사용되었고, Text-only NLE 로도 넘어오고 있다고 한다. (text-only 쪽이 상대적으로 더 어렵다고 한다.) 아직까지 이 분야에 적합한 데이터셋이 별로 없다고 한다. (대표적으로는 e-SNLI 정도?) 또한 explanation을 자연어로 생성해서 제공하는데, 동일한 현상에 대한 설명도 정말 다양하기 때문에 BLEU와 같이 NLG 에서 많이 사용되는 automatic metric을 이용해도 적절한 explanation을 검증하기가 쉽지 않다.
- e-SNLI: Natural Language Inference with Natural Language Explanations.
- Make Up Your Mind! Adversarial Generation of Inconsistent Natural Language Explanations.
- Rationalization: A neural machine translation approach to generating natural language explanations.
- Generating visual explanations.
- Generating Counterfactual Explanations with Natural Language.
- NILE : Natural Language Inference with Faithful Natural Language Explanations.
- Program Induction by Rationale Generation: Learning to Solve and Explain Algebraic Word Problems.
Probing
사실 제일 관심 있었던 부분은 이 섹션이다. Probing을 모델 내부에 학습된 정보를 확인하는 Task이다. 모델이 생성한 representation을 classifier의 입력으로 사용해서 성능을 측정하는데, downstream task에 적합한 정보다 더 많다면 더 좋은 representation을 생성해서 더 효과적인 classifier를 학습할 수 있다. 임베딩을 probing 하기도 하고, PLM 을 probing하기도 한다. Probing을 통해서 lower layer들을 fine-grained word-level syntactic information을 학습하고, higher layer emfdms global, abstract information 을 학습한다는 사실을 확인하기도 했다.
- What do Neural Machine Translation Models Learn about Morphology?.
- Evaluating Layers of Representation in Neural Machine Translation on Part-of-Speech and Semantic Tagging Tasks.
- What Does BERT Look at? An Analysis of BERT’s Attention.
- What you can cram into a single $&!#* vector: Probing sentence embeddings for linguistic properties.
- What is one grain of sand in the desert? analyzing individual neurons in deep nlp models.
- Probing for semantic evidence of composition by means of simple classification tasks.
- Under the Hood: Using Diagnostic Classifiers to Investigate and Improve how Language Models Track Agreement Information.
- A Tale of a Probe and a Parser.
- Designing and Interpreting Probes with Control Tasks.
- A Structural Probe for Finding Syntax in Word Representations.
- Multimodal explanations: Justifying decisions and pointing to the evidence.
- Spying on Your Neighbors: Fine-grained Probing of Contextual Embeddings for Information about Surrounding Words.
- Open Sesame: Getting inside BERT’s Linguistic Knowledge.
- Dissecting Contextual Word Embeddings: Architecture and Representation.
- Information-Theoretic Probing for Linguistic Structure.
- An Analysis of Encoder Representations in TransformerBased Machine Translation.
- Does String-Based Neural MT Learn Source Syntax?
- Firearms and Tigers are Dangerous, Kitchen Knives and Zebras are Not: Testing whether Word Embeddings Can Tell.
- Probing for Referential Information in Language Models.
- What do you learn from context? Probing for sentence structure in contextualized word representations.
Evaluation
평가 방식은 다양한데, 몇 가지만 나열해보면 :
1. precision score
2. faithfulness score : 입력에서 중요한 요소가 제외된다면 정답 class의 확률값이 크게 떨어진다는 사실을 기반으로 한다. 유사하게 그냥 중요한 정보를 제외했을 때, 성능(accuracy 등)이 떨어지는 정도를 보기도 한다.
3. BLEU score
4. Human Evaluation : 사람이 직접 평가하기 때문에 상대적으로 주관적인 평가를 하게 된다.
NLE에서는 설명을 생성하는 방식이기 때문에 NLG에서 많이 사용하는 metric인 BLEU, METEOR, ROUGE, CIDEr, SPICE 등을 사용하고, perplexity도 종종 사용된다고 한다. NLG에 포함된 task이기 때문에 human evaluation도 매우 중요하다.
* 추가적으로, 참고하면 좋을 것 같은 블로그 : https://christophm.github.io/interpretable-ml-book/
댓글